Ethical Data Analytics III – Solving an ethical use case
- alissahilbertz
- Sep 21, 2021
- 5 min read
Updated: Mar 29, 2024
In our ethical data analytics blog series, we’ve examined the potential ethical issues that can arise from data-driven decision making; how these issues can affect business, society, and consumers, and how to avoid them. In this last installment, we’re going to take a deeper look at how to go about eliminating or reducing ethical risks in data use cases.
The IG&H Ethical Risk Quick Scan is a tool for quickly assessing the ethical risk of a use case before a project is underway. The Quick scan is designed specifically to be useful in the early phases when many aspects of the final solution and model are not yet known. This way, ethical risk and complexity can be considered in use case prioritization and project approach.
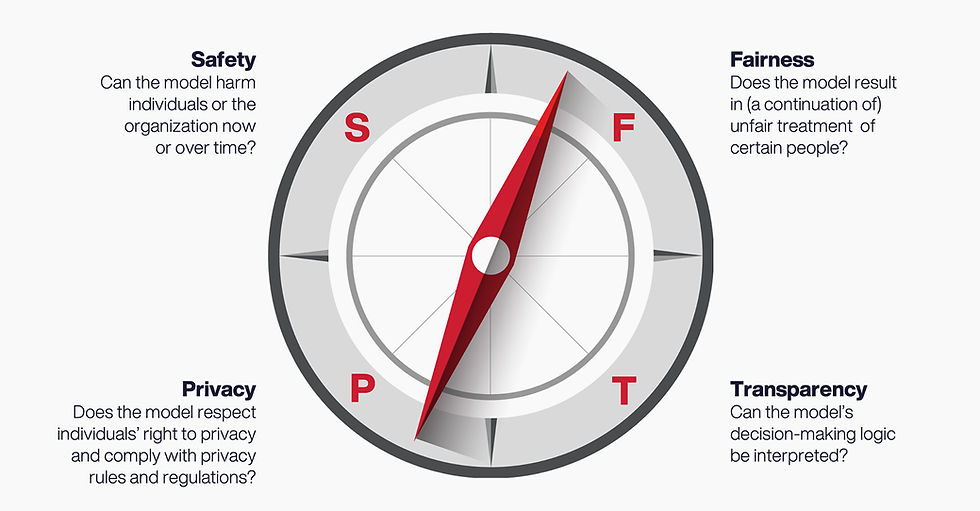
However, once a project is underway, the team will gain a better understanding of the data, the model workings, and the implementation requirements. At this point, the evaluation can be taken to the next level with the IG&H Ethical Guidance framework. This provides evaluation dimensions, more detailed check questions and possible solutions.
Our framework is based on four guiding dimensions and serves to inform each team’s moral compass [1]. Compared to the Quick Scan, the Guidance Framework is best used continuously throughout the project lifecycle, while more characteristics of the model and implementation become known.
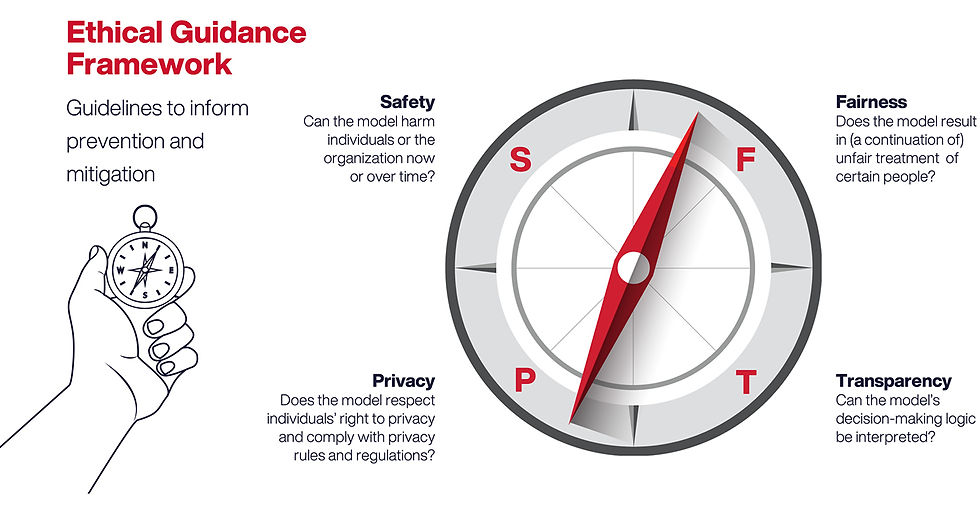
We loaded each dimension with sample check questions and several examples (of many) of the ways in which risks can be prevented or mitigated [2]. We will first illustrate the application of the framework with a use case and then provide a table with the check questions and solution examples after this case.
Ethical guidance in action: taxi company use case
We’re now going to apply this framework in a realistic but virtual use case. Let’s take the example of the taxi service that digitally monitors many aspects of the rides assigned to their drivers. The company wants to develop a model that will score driver performance and automatically adjust driver paychecks accordingly. Please note that while this example is representative of work we have done for different clients, it is not an actual client use case.
When we applied the Quick scan before the project started, six of the eight categories in the Quick Scan signaled medium to high risk. It is no surprise then that when we built this automated scoring and decisioning model [3] for the company, we ran into some ethical challenges. We evaluated the project using the Guidance Framework monthly and the identified challenges all fall within (at least) one of the topics in the framework.
Safety
The Quick Scan already warned us that the intent to have no human in the loop is a risk factor, particularly because the model may affect matters of life. This means that if the model accuracy degrades over time (model drift), or it produces unintended outputs due to data quality issues, no one might notice before the drivers are impacted. Considering the ‘Safety’ dimension of our Guidance framework, we choose to perform two mitigative actions: sensitivity analysis before deployment and the implementation of statistical monitoring of the model’s inputs and outputs.
Fairness
In the Quick Scan, we indicated a medium risk for bias in the data, meaning that we might need extra effort to ensure ‘Fairness’ per our Guidance framework. When we analyzed the model test results, we found that female driver scores were skewed toward the higher end of the scale and male driver scores towards the lower end [4].
At this point, we needed to understand whether the model was not accidentally biased with respect to gender in its scoring [5]. After careful examination, we conclude that the model is using valid, objective features for its scoring. And that female and male drivers receive the same scores for the same driving behaviors. So, together with the taxi company we decide no change is needed, but this analysis is documented for future reference.
Transparency
As we saw in the Quick Scan, affecting matters of life was a risk factor for this use case. Matters of life includes things that may impact a person’s quality of life. For example, in financial services, this might be the affordability of credit, or in healthcare, it might be access to certain treatments. For the taxi company, the model’s decisions will certainly impact employees financially through their paychecks.
The model may also influence personal behavior, which we considered a medium risk in this case. For example, a driver may feel pressured to drive through more dangerous neighborhoods or accept rides from individuals who might pose a threat.
As one of our mitigative actions we must provide a clear explanation to the taxi drivers how the model works. We also introduce a process to handle any questions from drivers going forward. Finally, together with the model owners and HR we also ensure that the model’s impact on rewards distribution and driving behavior will be monitored. This way, the taxi company can provide the transparency that is justified by the potential impact of this model.
We would also like to mention here that there should always be a formal company representative who is accountable for a decision model’s impact on people and business. In this case, the COO was assigned as the accountable model owner at the start of the project [6].
Privacy
The taxi company’s dataset contained geo-location data that could be used to (re)identify individuals once combined with other, publicly available data. Other sensitive personal data elements such as ‘gender’ and ‘age’ were kept from the model to avoid unwanted bias, but we did keep these in our dataset to be able to test the model’s fairness. The employees should be able to trust that their privacy is respected and that their personal data is processed and stored securely. Therefore, we perform a Data Privacy Impact Assessment and apply all the company’s technical and operational measures to ensure its protection.
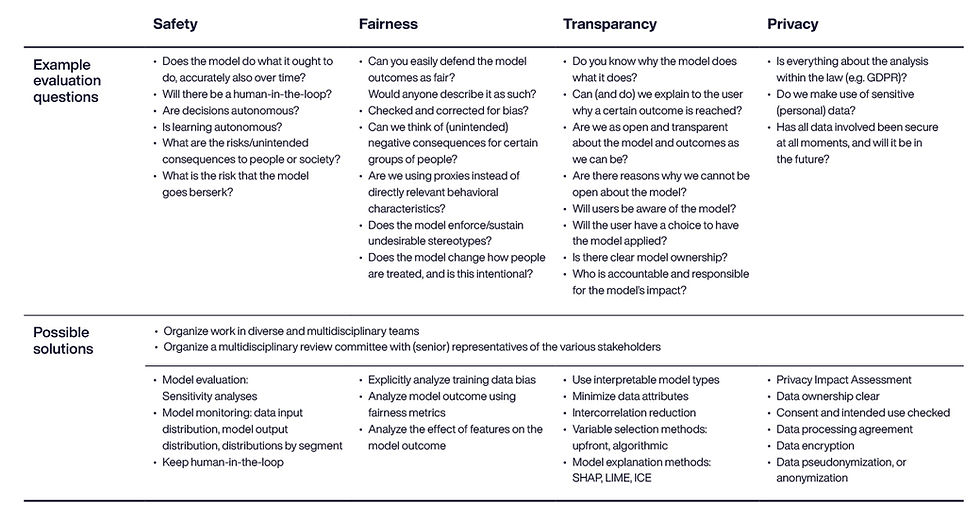
IG&H Ethics-centricity
The case above shows that if you keep data ethics top-of-mind, many potential issues can be avoided or mitigated. At IG&H we put ethics right next to economics at the center of everything we do. And we know our clients care as much about this as we do. Striking a balance between these two seemingly opposing forces is one of the many fun and fascinating aspects of our daily work.
Since the ethics of a data project are perhaps a bit fuzzier than the economics, we created our frameworks as a guide. Why? To provide the needed structure and early warning signals that help our clients follow their moral compass and take adequate measures. This way, everyone can enjoy the benefits of data and AI safely and fairly.
Authors
Tom Jongen
Floor Komen
[1] The SFTP guidelines were distilled by IG&H from a meta study done on 36 prominent AI principles documents.
[2] Let us mention here that the field of data ethics is rapidly evolving, and so is our framework. When we encounter new relevant topics, more check questions and solutions are added.
[3] Such a model would be denoted a ‘High risk AI system’ in upcoming AI regulation, which will carry extra responsibilities in the future.
[4] We did not use the variable ‘gender’ in the model itself but did keep it in the dataset for validation purposes. This way, sensitive data may be collected for a model, but exactly for the purpose of protecting against violation of this sensitivity.
[5] Even if inequality in a model is not the result of bias, you might have good reason to eliminate the inequality. We chose not to cover this in this article, but bias/inequality might be a good topic for an article on its own.
[6] The COO is accountable for the model’s impact on business and people, and she had delegated the day-to-day responsibility for this to the company’s performance manager.