Tailored Trends: A Data-Driven Approach to Fashion Assortments
- alissahilbertz
- Dec 12, 2023
- 7 min read
Updated: Feb 5, 2024
Fashion has inherently complex business models. These are proving to be unsustainable, leading to vast piles of overstock, for example. Kristel Lanjouw, Head of Data & Analytics at IG&H, describes how data can help reshape the industry for the better.
Fashion trends shift rapidly, seasons change by the minute. What is considered hot one season can quickly become passé in the next. Disposable clothing, designed for constant consumer turnover, also takes a heavy toll on the environment. Fashion is one of the world's most polluting industries, many workers face exploitation and the aftershocks of the COVID-19 pandemic add to this burden. Yet, fashion presses on with its inherently complex and unsustainable business models.
Although this creativity-driven sector is notoriously data-averse, e-commerce is one area that leverages data. Think of your online shopping cart or wish list. Chances are that some items made their way in there because of product recommendations or personalized ads. Algorithms learn about us and cater to our preferences. On the one hand, this democratizes the creation of trends. After all, millions of people searching online for cowboy boots are difficult to ignore. On the other hand, the influx of information from social media, style blogs and co. provides brands with an unprecedented number of clues and insights into their consumers' desires and behavior.
Despite all this available data the industry still relies heavily on intuition and opportunism in creating collections. This leads to a common issue: excessive overstock at the end of each season. Overstock easily reaches fifty percent, which ultimately needs to be dissolved by markdowns that appear earlier and more frequently each year. How can fashion brands escape this tendency to overstock and reshape the industry for the better?
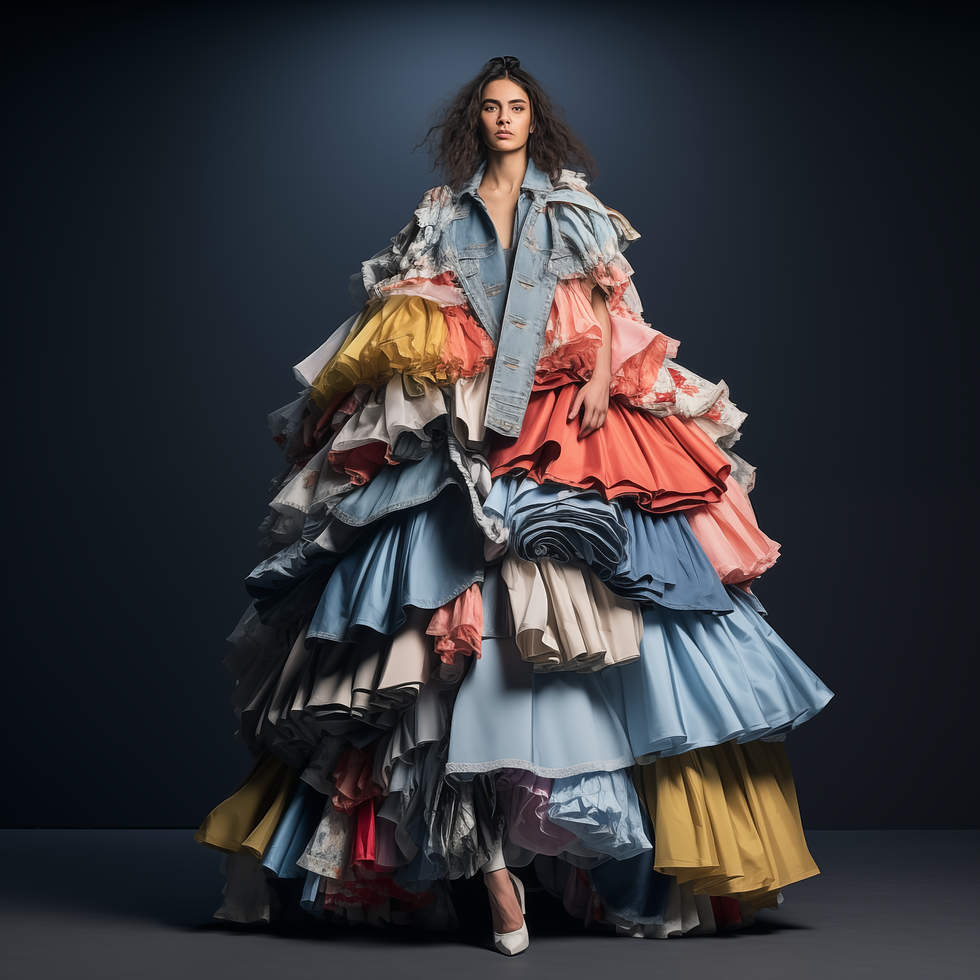
A winter coat made from the season's overstock, created with Midjourney
Endless opportunism or data-driven assortment management?
The prevailing mantra for sales teams has been "better to overstock than to understock'', further escalated by endless opportunism of the creatives and commercials: “This flared denim will sell like hotcakes!” However, this unsustainable model based on quality and experience of the designers, planners and sellers has taken a toll on both the environment and operational costs. A general "more, more and more just in case" is not future proof.
Instead, fashion companies must strive for precision in product placement. Currently, they scatter the same items across diverse locations around the globe. It doesn't take a PhD in Design to know that each country has its own tastes and spending power or that the weather in Barcelona is warmer than in Stockholm. However, shelves are bursting with the same amount of summer shorts in Spain as in Sweden. Why is it that brands still operate in this old-fashioned “one-size-fits-all” model? How can brands better tap into local consumer demands?
The answer is data-driven assortment management. With this approach, a brand knows what its consumers want to buy bottom-up (local point of sale), and via which channels, based on taste, weather, but also demographics, spending power, etc.
Cost of complexity vs. value of variety
The challenge is balancing the increasing operational costs against the value of providing more diverse local assortments. Before you know it, data-driven assortment management becomes extraordinarily complex. In its most extreme form, you would optimize every single point of sale (PoS). Each PoS is unique, with unique customers that have their unique needs... optimizing each one won't work, at least not if profit is one of your KPIs. Data-driven assortment management offers a solution. It allows you to fine-tune product offerings to match local demand, achieving a delicate balance between standardization and regional differentiation. The result? Typically, a three to five percent gross margin uplift for both small and large fashion brands.
This approach thrives on data analytics, customer behavior insights and regional nuances. For instance, it recognizes that Scandinavians lean towards dark, plain colors, while Spanish consumers favor prints and brighter shades for their blouses. Or that Eastern European consumers need two extra seasons before picking up on the German trends. It also understands that consumers prefer buying bathrobes online instead of going to the store to try them on. The model evolves and self-learns over time, so it only becomes better. It can also include strategic growth areas. For instance, a brand may want to become better known for its men's polo shirts. The model can advise on how to increase specific designs and textures in which locations.
Blending creativity with data
Fashion is volatile, unpredictable and ultimately an emotional product. Whether it's a power suit, a statement piece you found at a thrift store or a completely new look, you choose what you communicate to the outside world. Data-driven category management doesn't strip fashion of its artistry. Instead, it offers a systematic approach.
Machine learning continually refines the model, learning from past seasons. Buyers and designers can also input their expertise and preferences, making it a collaborative and predictive tool. With data to get it right and technology to make it work, the best guess is no longer the only option. Algorithms won’t bring us perfection. However, teasing out the best combination of people, data and tech may well be the new art of the increasingly digital fashion world.
Case study #1
Fashion Brand Unravels the Science of Attribute Combinations
A large international fashion brand faced a huge challenge in the fashion retail battleground. Following a 'one-size-fits-all' assortment strategy, they offered the same products across their European stores, irrespective of location. This approach led to the audacious move of introducing winter coats in the Mediterranean's scorching heat and fresh sundresses in the icy North.
In their quest to enhance profitability and local appeal, they teamed up with IG&H to conduct a profound data analysis in one of their key markets. They had been losing significant market share for a couple of seasons already.
Blending data and retail insights allowed us to uncover hidden patterns in product performance. In fashion assortments, the key is a detailed understanding of the winning combinations of different attributes, on a product category level. For example: A blue, long-sleeved, cashmere men’s sweater with a round neck sold tremendously better than its V-neck counterpart. Cotton wrap-dresses with an all-over print always sold out in sizes L and XL. Neon colors were a favorite in the youngsters’ section, specifically bright shirts and skirts. For shorts, organic fibers combined with soft, neutral and pastel colors proved to be an absolute bestseller.

Using a Key Performance Indicator (KPI) to gauge relative product profitability within a category, we pinpointed the attribute combinations that consistently outperformed their goals and those that fell short.
How were these results implemented?
Pre-season
Specific recommendations on their next seasonal buy: attribute combinations were mapped out, buying volume variations on SKU-level were suggested.
Mid-season
During the season, recommendations on replacing underperforming items with attractive proven alternatives were made: coming from different stores, channels or countries.
Post-season
The model checked if the predicted recommendations were indeed correct, and where they could be improved, helping to re-iterate the algorithm for the next season.
Results
By leveraging machine learning on an ongoing basis, we realized a ~5% profit margin increase, accompanied by a qualitatively recognized increase in local brand relevance. Furthermore, the model was applied in all other European markets.
Case study #2
Footwear retailer embraces E2E planning with our partner o9 Solutions
A globally renowned casual footwear leader had significant growth perspectives. However, it also faced a long time to market and very manual pre-season and in-season planning. Optimizing assortment and inventory planning was particularly challenging for three reasons: First, the customers’ continuous desire for newness. Second, the long time to market. Third, the poor forecast accuracy that resulted from a time-consuming manual process prone to crashing spreadsheets. As a result, the company's business was booming, but the planning infrastructure struggled to keep up.
The company has chosen our technological partner o9 Solutions to build more compelling assortments for each sales channel and geography while better-managing inventory levels, reducing planning cycles and improving forecast accuracy all on one platform in one integrated flow.
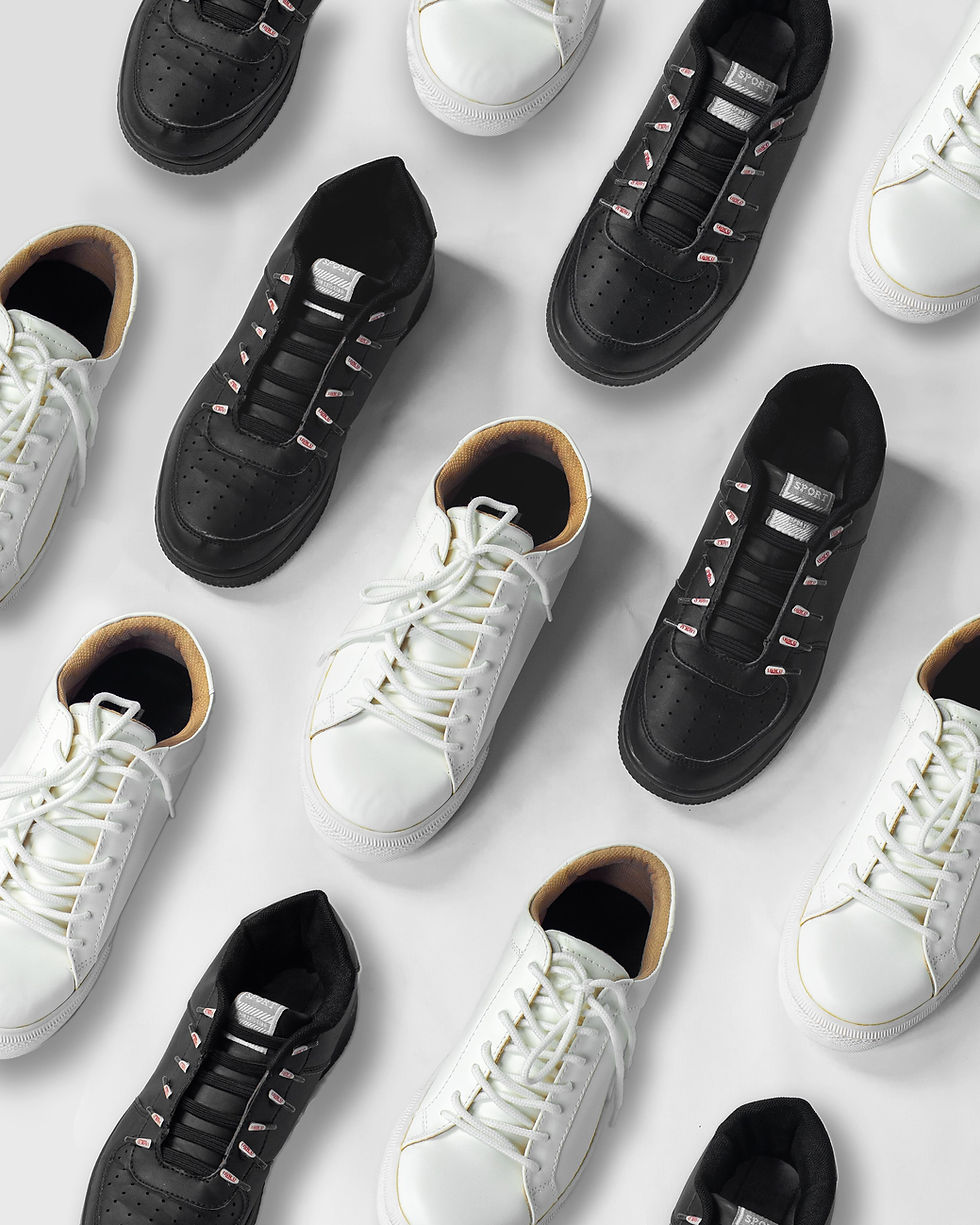
How were these results implemented?
o9’s platform enabled end-to-end pre-season and in-season planning, covering:
Merchandise Financial Planning (MFP): top-down and bottom-up reconciliated multi-currency financial targets (sales, margin, discount and returns) by merch segment and channel using attribute and scenario planning, framing demand early on for an adequate supply response.
Assortment planning (AP): Global and regional pre-season assortment plan connected with the MFP plan modeling for in-line / out-of-line and new product introductions/carryover/core products, using attribute and style-color ranking and size tiering to ensure a tailored approach to diverse markets.
Demand planning (DP): One global and more accurate demand plan defined by style, sub-region, sub-channel for all merch segments and feeding Merchandise Financial Planning, Assortment planning, OTB and Supply planning.
Open To Buy (OTB): An in-season monthly sales and inventory plan at Sub-region/inventory segment level for retail and wholesale channels with rolling 24 months to drive inventory decisions and reduce waste and stock outs.
Supply and Inventory Planning: one supply plan at material and SKU level driving replenishment, stock rebalancing and buying decisions in accordance with the Demand Plan priorities and ultimately optimizing inventory levels and cost.
The retailer witnessed a 51% increase in forecast accuracy, a staggering 10x improvement in planner efficiency leading to a reduction in planning cycle times, and a shift from 39 databases to a unified platform. These results ultimately enabled better control over inventory levels, reduced lost sales due to improved demand signals and a more responsive go-to-market strategy.
Your partner in data-driven fashion
With over 35 years of retail experience, we understand the fashion industry's complexity sets it apart. You can rely on IG&H as a sector insider who has all the necessary capabilities for a smart, data-driven way of working. Our select technology partners and in-house developers allow us to build bespoke and off-the-shelf solutions for you.
Are you ready for data to benefit both your business and consumers? We bring all necessary capabilities together and ensure your new strategy lands with your organization, fast-tracking your fashion transformation. Learn more about how you can use a data-driven approach to delight consumers and run a smarter race. Get in touch:
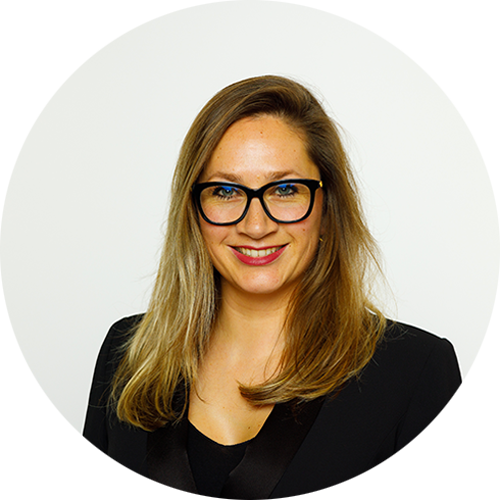
Kristel Lanjouw
Head of Data & Analytics
T: +31 625493984